The "Multi-FoV" synthetic datasets
We provide two synthetic scenes (vehicle moving in a city, and flying robot hovering in a confined room). For each scene, three different optics were used (perspective, fisheye and catadioptric), but the same sensor is used (keeping the image resolution constant). These datasets were generated using Blender, using a custom omnidirectional camera model, which we release as an open-source patch for Blender. The Blender scene for the urban canyon dataset was generated using the addon Scene City. The Blender file is available here. The Blender scene used for the room environment can be found here.
The camera calibrations, groundtruth trajectories and depth maps are provided in the archives. For any question relative to these datasets, please send an e-mail at rebecq (at) ifi (dot) uzh (dot) ch .
![]() |
![]() |
![]() |
Perspective | Fisheye | Catadioptric |
Perspective (with depth maps) | Fisheye (with depth maps) | Catadioptric (with depth maps) |
Ground truth | Ground truth | Ground truth |
![]() |
![]() |
![]() |
Perspective (with depth maps) | Fisheye (with depth maps) | Catadioptric (with depth maps) |
Ground truth | Ground truth | Ground truth |
License
This dataset and the Blender patch are released under the Creative Commons license (CC BY-NC-SA 3.0), which is free for non-commercial use (including research).References
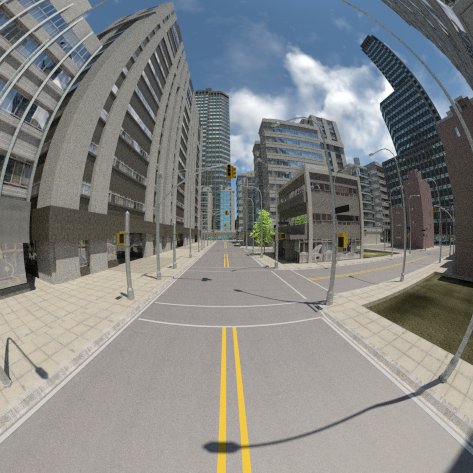
Benefit of Large Field-of-View Cameras for Visual Odometry
IEEE International Conference on Robotics and Automation (ICRA), Stockholm, 2016.
PDF YouTube Research page (datasets and software) C++ omnidirectional camera model
Motivation: Study the influence of field-of-view on visual odometry
The transition of visual-odometry technology from research demonstrators to commercial applications naturally raises the question: "what is the optimal camera for vision-based motion estimation?" This question is crucial as the choice of camera has a tremendous impact on the robustness and accuracy of the employed visual odometry algorithm.
While many properties of a camera (e.g. resolution, frame-rate, global-shutter/rolling-shutter) could be considered, in this work we focus on evaluating the impact of the camera field-of-view (FoV) and optics (i.e., fisheye or catadioptric) on the quality of the motion estimate. Since the motion-estimation performance depends highly on the geometry of the scene and the motion of the camera, we analyze two common operational environments in mobile robotics: an urban environment and an indoor scene.
To confirm the theoretical observations, we implement a state-of-the-art VO pipeline that works with large FoV fisheye and catadioptric cameras. We evaluate the proposed VO pipeline in both synthetic and real experiments. The experiments point out that it is advantageous to use a large FoV camera (e.g., fisheye or catadioptric) for indoor scenes and a smaller FoV for urban canyon environments.