The Event-Camera Dataset and Simulator:
Event-based Data for Pose Estimation, Visual Odometry, and SLAM
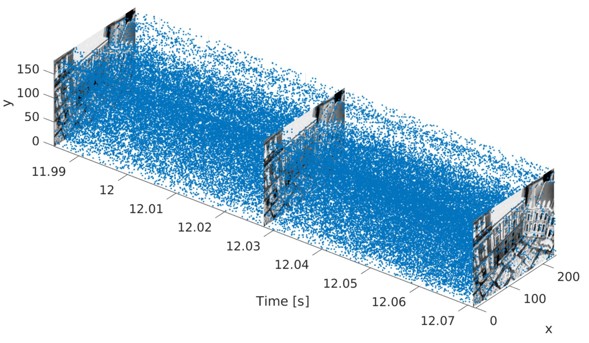
This presents the world's first collection of datasets with an event-based camera for high-speed robotics.
The data also include intensity images, inertial measurements, and ground truth from a motion-capture system.
An event-based camera is a revolutionary vision sensor with three key advantages:
a measurement rate that is almost 1 million times faster than standard cameras,
a latency of 1 microsecond,
and a high dynamic range of 130 decibels (standard cameras only have 60 dB).
These properties enable the design of a new class of algorithms for high-speed robotics, where standard cameras suffer from motion blur and high latency.
All the data are released both as text files and binary (i.e., rosbag) files.
Links:
More on event-based vision research at our lab
Tutorial on event-based vision
The Event-Camera Dataset and Simulator: Event-based Data for Pose Estimation, Visual Odometry, and SLAM
International Journal of Robotics Research, Vol. 36, Issue 2, pages 142-149, Feb. 2017.
We provide all datasets in two formats: text files and binary files (rosbag). While their content is identical, some of them are better suited for particular applications. For prototyping, inspection, and testing we recommend to use the text files, since they can be loaded easily using Python or Matlab. The binary rosbag files are intended for users familiar with the Robot Operating System (ROS) and for applications that are intended to be executed on a real system. The format is the one used by the RPG DVS ROS driver. The details about both format follows below.
The format of the text files is as follows.
The rosbag files contain the events using dvs_msgs/EventArray message types. The images, camera calibration, and IMU measurements use the standard sensor_msgs/Image, sensor_msgs/CameraInfo, and sensor_msgs/Imu message types, respectively. Ground truth is provided as geometry_msgs/PoseStamped message type.
We provide various plots for each dataset for a quick inspection. The plots are available inside a ZIP file and contain, if available, the following quantities:
These datasets were generated using a DAVIS240C from iniLabs. They contain the events, images, IMU measurements, and camera calibration from the DAVIS as well as ground truth from a motion-capture system. The datasets using a motorized linear slider neither contain motion-capture information nor IMU measurements, however ground truth is provided by the linear slider's position. All datasets in gray use the same intrinsic calibration and the "calibration" dataset provides the option to use other camera models.
Name | Motion | Scene | Download | Refs |
shapes_rotation | Rotation, increasing speed |
Simple shapes on a wall |
Text (zip) rosbag Plots (zip) |
[4] |
shapes_translation | Translation, increasing speed |
Simple shapes on a wall |
Text (zip) rosbag Plots (zip) |
[5], [6], [7] |
shapes_6dof | 6-DOF, increasing speed |
Simple shapes on a wall |
Text (zip) rosbag Plots (zip) |
[5], [6], [7] |
poster_rotation | Rotation, increasing speed |
Wallposter |
Text (zip) rosbag Plots (zip) |
[3], [4] |
poster_translation | Translation, increasing speed |
Wallposter |
Text (zip) rosbag Plots (zip) |
[5], [6], [7] |
poster_6dof | 6-DOF, increasing speed |
Wallposter |
Text (zip) rosbag Plots (zip) |
[5], [6], [7] |
boxes_rotation | Rotation, increasing speed |
Highly textured environment |
Text (zip) rosbag Plots (zip) |
[3], [4] |
boxes_translation | Translation, increasing speed |
Highly textured environment |
Text (zip) rosbag Plots (zip) |
[5], [6], [7] |
boxes_6dof | 6-DOF, increasing speed |
Highly textured environment |
Text (zip) rosbag Plots (zip) |
[5], [6], [7] |
hdr_poster | 6-DOF, increasing speed |
Flat scene, high dynamic range |
Text (zip) rosbag Plots (zip) |
[5], [6], [7] |
hdr_boxes | 6-DOF, increasing speed |
Boxes, high dynamic range |
Text (zip) rosbag Plots (zip) |
[5], [6], [7] |
outdoors_walking | 6-DOF, walking |
Sunny urban environment |
Text (zip) rosbag Plots (zip) |
|
outdoors_running | 6-DOF, running |
Sunny urban environment |
Text (zip) rosbag Plots (zip) |
|
dynamic_rotation | Rotation, increasing speed |
Office with moving person |
Text (zip) rosbag Plots (zip) |
[3], [4] |
dynamic_translation | Translation, increasing speed |
Office with moving person |
Text (zip) rosbag Plots (zip) |
[5], [6], [7] |
dynamic_6dof | 6-DOF, increasing speed |
Office with moving person |
Text (zip) rosbag Plots (zip) |
[5], [6], [7] |
calibration | 6-DOF, slow |
Checkerboard (6x7, 70mm), for custom calibrations, only applies to above datasets in gray |
Text (zip) rosbag Plots (zip) |
|
office_zigzag | 6-DOF, zigzag, slow |
Office environment |
Text (zip) rosbag Plots (zip) |
[1] |
office_spiral | 6-DOF, spiral, slow |
Office environment |
Text (zip) rosbag Plots (zip) |
[1] |
urban | Linear, slow |
Urban environment |
Text (zip) rosbag Plots (zip) |
[1] |
slider_close | Linear, constant speed |
Flat scene at 23.1 cm |
Text (zip) rosbag Plots (zip) |
[2] |
slider_far | Linear, constant speed |
Flat scene at 58.4 cm |
Text (zip) rosbag Plots (zip) |
[2] |
slider_hdr_close | Linear, constant speed |
Flat scene at 23.1 cm, HDR |
Text (zip) rosbag Plots (zip) |
[2] |
slider_hdr_far | Linear, constant speed |
Flat scene at 58.4 cm, HDR |
Text (zip) rosbag Plots (zip) |
[2] |
slider_depth | Linear, constant speed |
Objects at different depths |
Text (zip) rosbag Plots (zip) |
[2] |
We provide two synthetic scenes. These datasets were generated using the event-camera simulator described below.
Name | Motion | Scene | Download | Refs |
simulation_3planes | Translation, circle | 3 planes at different depths |
Text (zip) rosbag Plots (zip) |
[2] |
simulation_3walls | 6-DOF | Room with 3 textured walls |
Text (zip) rosbag Plots (zip) |
[2] |
Low-Latency Visual Odometry using Event-based Feature Tracks
IEEE/RSJ International Conference on Intelligent Robots and Systems (IROS), Daejeon, 2016.
EMVS: Event-based Multi-View Stereo
British Machine Vision Conference (BMVC), York, 2016.
Accurate Angular Velocity Estimation with an Event Camera
IEEE Robotics and Automation Letters (RA-L), Vol. 2, Issue 2, pp. 632-639, Apr. 2017.
Real-Time Panoramic Tracking for Event Cameras
International Conference on Computational Photography (ICCP), May 2017.
Best Paper Award
Event-based Visual Inertial Odometry
Computer Vision and Pattern Recognition (CVPR) 2017.
Real-time Visual-Inertial Odometry for Event Cameras using Keyframe-based Nonlinear Optimization
British Machine Vision Conference (BMVC), London, 2017.
Ultimate SLAM? Combining Events, Images, and IMU for Robust Visual SLAM in HDR and High Speed Scenarios
IEEE Robotics and Automation Letters (RA-L), 2018.